AI, can I get a second opinion?
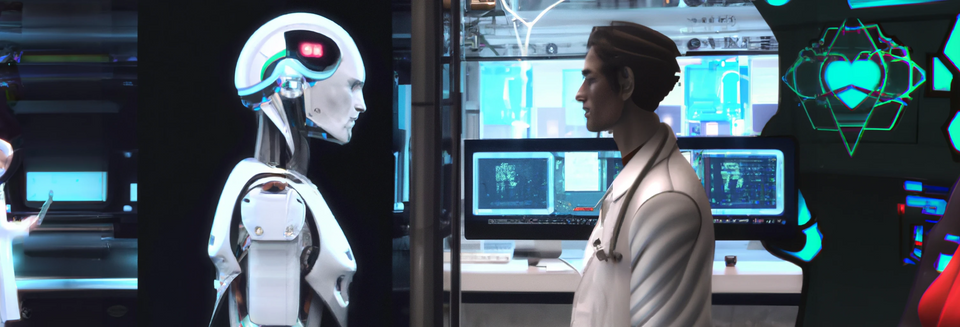
The state-of-play in medical AI
Have you ever walked out of a GP or physician consult struck by the lack of quality data, insights and software at their disposal? I know I have (repeatedly!), and I joined the Antler Amsterdam residency in May 2023 to see if I could do something about it. The fact of the matter is, doctors far too often have no clue whether or not their prescriptions will work for a specific patient — and no way of finding out. As a result, their decisions about your treatment plan will be based on their professional experience, education, what they learn from peers, and the promotional material pharmaceutical companies are pushing their way. To me, this doesn’t seem right given all that we can already do with AI. What is worse, the diagnoses they make are wrong more often than you’d care to know. Lacking good quality data on medical diagnostic errors (for reasons we’ll dive into below), a cursory glance at a recent study comparing GPT4 versus human ophthalmologists on a diagnostic question answering task showed that where GPT4 got 73% of the questions correct, team human scored a meagre 58%! Those are pretty bad odds — imagine every flight you take you only has an 58% chance of arriving at the correct destination! In fact, this is only just slightly higher than my I’m-going-to-wing-it-zero-effort high school final exam GPA of 57%.
It’s not all negative of course. Modern medicine has seen some amazing advances, and there are quite a few things Western healthcare systems have gotten nailed down to a tee. In fact, many of the structural issues are being addressed by a mix of healthcare professionals, startups, lawmakers, institutions and incumbent industry players — unfortunately, the healthcare space being as regulated, complex and opaque as it is, most of these changes are happening at a snail’s pace. I want to walk you through three of the these structural issues, and discuss some of the solutions being worked on. Hopefully after reading this post, next time you attend a consult you will be better able to identify the red flag systemic issues at your care provider. This should — in theory at least :) — help you weigh your doctor’s words a little better, helping you make more informed choices about the treatment plan.
Problem #1: A broken healthcare system
As I’m sure a lot of you will have experienced, medical care in the Western world can come across as quite dehumanising. Large teams of healthcare professionals are involved when the procedures are complex or take more than two hours, and individual decisions quickly get lost in a whirlpool of (digital) paperwork and handovers between departments, teams and individual team members. Two very real and noticeable knock-on effects of this way of working are the reduction of agency of individual healthcare (HC) professionals and a massive increase in administrative tasks. HC professionals are spending evenings and weekends trying to get their administration in order because their daily schedule often leaves them with insufficient time to complete these tasks during working hours.
Unsurprisingly, this has led to significant decreases in job satisfaction and higher rates of burnout among HC professionals. Not to mention that we as a society are pushing the very real costs of our current healthcare systems into the private lives of HC professionals — they are the ones who pay for this by spending less time with their family or seeing less patients (and therefore earning less money) in case of a private practice.
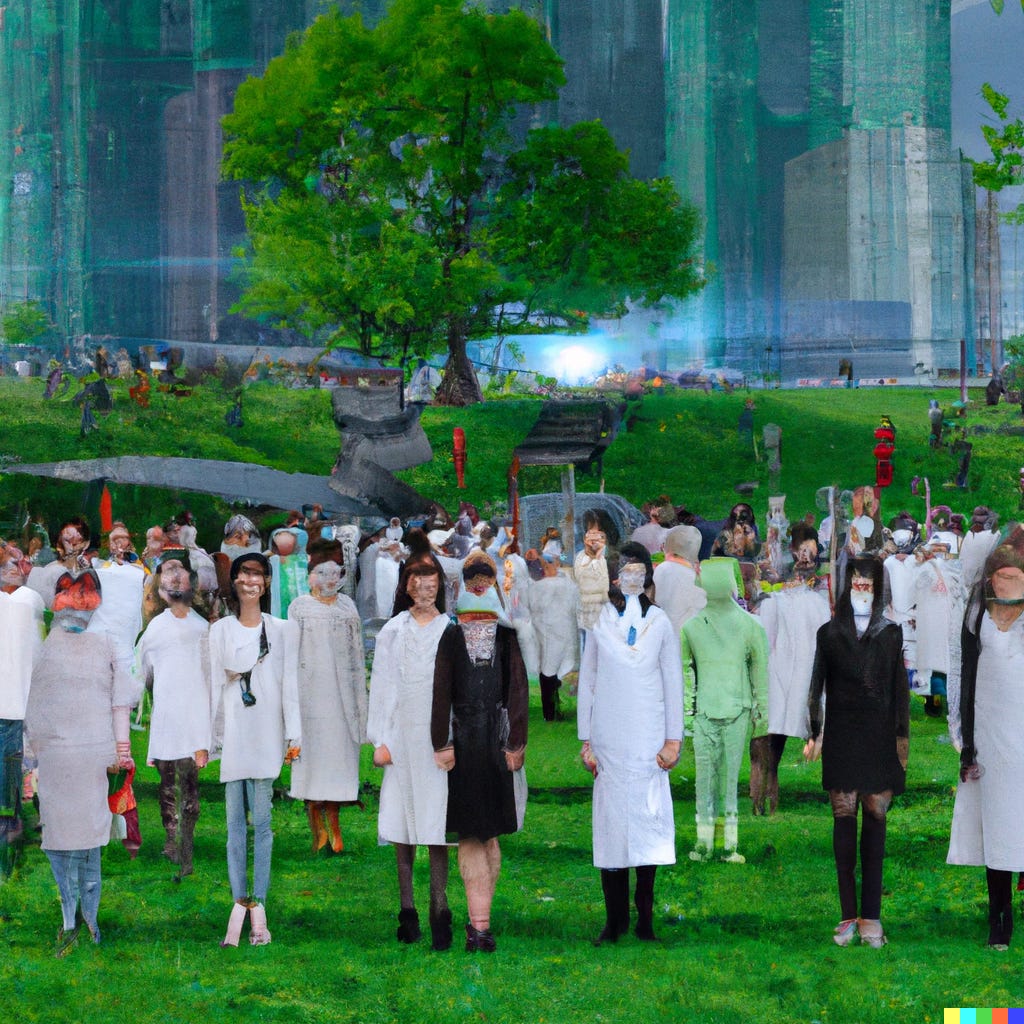
There are many reasons why the administrative burden has increased. From the outside looking in, the two primary suspects are the institutionalisation of medicine and the way healthcare IT systems are implemented. Large hospitals with siloed departments facilitating the different branches of medicine naturally come with an enormous communication and administration overhead. Factor in shifts (handovers between HC professionals with the same expertise), legal requirements, healthcare insurer demands, and complex procedures involving different departments and it becomes easy to see why a GP in rural Virginia in the 1950s could do with a notebook, but the resident ophthalmologist in a 10,000-staff modern-day hospital is spending half her day on her hospital OS. Given the scale and time constraints in contemporary HC systems, in-person handovers are mostly done through Electronic Health Records (EHRs). They are the glue of our healthcare systems, and — again very much unfortunately — often myopic in their implementation. To this day (anno 2023) a lot of HC IT systems are solipsistic, facilitating a single hospital, GP practice, or other HC facility. There is a push for greater interoperability between systems by adopting data API standards like FHIRv5, but (again from the outside looking in) I get the impression that most healthcare IT systems are a draconian mess of customised software modules living on top of 1990s-style hard- and software with zero documentation, very little accountability and even less future-proofing.
Which is concerning to say the least, since these systems are the lifeblood of modern medicine, directly impacting life or death situations. We’ll dive into the knock-on effects for quality of care in the next section, but to lift some of the doom and gloom I wanted to discuss a number of initiatives addressing the two most prominent symptoms of our broken healthcare systems — decreases in HC professional productivity and agency.
Patient screening solutions
One of the hallmark medical AI problems is to reducing the number of unnecessary consults by automating patient screening. These solutions are typically bundled with functionality that automates low-risk actions that don’t necessarily require human intervention, such as fulfilling recurring subscriptions. Given that these are relatively low-impact problems, even an AI system operating at 95% accuracy could function quite well with significantly more upside than downside — as long as risks are managed correctly. Two notable startups focusing on this problem are:
- Ada Health. Established in 2017 in Germany, raised EUR 175M (USD 185M) to date with over 14M users, mostly in the US. Their main product is a symptom checker consumers can use to determine if they need to check in with their GP.
- Corti. Established in 2016 in Denmark, raised EUR 91M (USD 86M) to date. Their product suite focuses on augmenting first line responder tele-screening with AI, ensuring critical conditions that require direct interventions are detected more often.
In-consult productivity solutions
While it was possible to develop functional screening solutions in the pre-LLM era of ML, the rapid rise of novel AI models and methods these last few years has helped spur the rise of a new wave of in-consult solutions. This new wave, propelled by recent advances in NLP (GPT!) seems to have a lot better chance of reaching critical mass of user adoption. Some exciting examples of startups working on in-consult solutions focused on reducing the administrative burden of clinicians are:
- Navina. Founded in 2018 in the US, raised EUR 42M (USD 44M). Automated mining of EHR records for GPs. They claim their data visualisations reduce the time it takes GPs to prepare for a patient consult from 30m to 5m.
- Suki.ai. Founded in 2016 in the US, raised EUR 90M (USD 95M). AI voice assistant for in-consult medical note-taking, in-consult EHR information retrieval and in-consult medical question answering (mQA).
- Nabla. Founded in 2018 in France, raised EUR 20M (USD 21M). AI-powered automated clinical note taking app.
Problem #2: Good quality data is incredibly rare
There is a significant secondary-order impact on quality of care and patient outcomes of running healthcare with best-effort, locally optimised IT systems: they make it very hard to run independent large-scale studies of patient health and treatment effects. Because of this, pharmaceutical companies have been effectively given a monopoly on healthcare data — meaning they have to a degree become the sole guardians of truth. Their primary incentives being commercial in nature, more aligned with their shareholders than their wider group of stakeholders, this is not an ideal situation. It can even be argued that the lack of independent healthcare data collection at scale is one of the main culprits for the massive YoY increases in healthcare costs in the US, where pharmaceutical companies have been allowed to extract ridiculous amounts of rent from their IP with only the thinnest veil of scientific evidence that their products actually work.
Even beyond data ownership, there are also big questions that can be asked of the current methodology used to collect and process data as evidence for patient treatments. Given that pharmaceutical companies mainly collect data through the large-scale clinical trials needed to launch and register a new drug, very little data is available on the long-term effects and patient outcomes of individual drugs. In addition, these large-scale trials are mostly conducted with healthy (i.e. non-representative) trial participants. This means that a lot of drugs allowed onto the market have in effect not been properly tested. So next time you get a prescription, be aware that the evidence of its benefits is often sketchy (as in p-hacked or cherry-picked), outdated and incomplete at best, and that it is possible harmful side effects have been swept under a proverbial rug. This has led to a large number of cases (no one can say for sure how much, since there is no independent data collection in place) where clinicians have prescribed potentially harmful medication to patients — medication where for certain (sub)groups of patients the Number Needed to Treat (NNT) (the number of patients needed to produce one positive outcome) is actually significantly higher than the Number Needed To Harm (NNH), meaning that the odds that a patient experiences harmful side-effects from the medication are actually larger than the odds of the medication improving their condition. For me personally, finding that the ratio of NNT to NNH was often too close to call was one of the most unexpected findings of the research I did this summer, and one that severely damaged my trust in modern medicine.
But the worst thing is that even if there is good statistical evidence that the medication will work, selecting a treatment plan based on evidence from Randomised Controlled Trials does nothing to help the clinician predict how an individual patient will respond to a treatment. Fortunately, there is light at the end of the tunnel — two mega trends in medicine that I hope will steer us clear of the hopelessly inefficient and ineffective way of practicing medicine called ‘evidence-based medicine’:
- the rise of precision medicine, and
- a methodological push away from average treatment effects towards estimates of individual treatment effects using data and ML.
So things are bad — try to stay out of a hospital for the next 20 years if you can — but will get better eventually. Advances in precision medicine will allow the development of personalised therapeutics, tailored to our individual genomes and biomes. Rapid technological advances (such as DeepMind’s AlphaFold) coupled with massive decreases in drug development costs are still needed to make these kind of therapeutics a practical option, but big steps are being taken each year and linear (or hopefully exponential) progress will get us there sooner rather than later. Fuelled by advances in AI, AI drug discovery startups have become a key area of focus for VC and other investment in medical AI in recent years.
While the efficiencies of scale for precision medicine are still a few years away, there are steps that can be — and are being — taken by practitioners today to deal with some of the shortcomings in the current medical practice. These steps include better (independent) data collection, better collaboration between HC institutions, and methodological improvements in estimating treatment effects with statistical and machine learning methods such as the Individualised Treatment Effect (ITE) illustrated below. The efficacy of these machine learning methods rely on the quality and volume of data available, so any steps taken in this direction will need to start with better data collection.
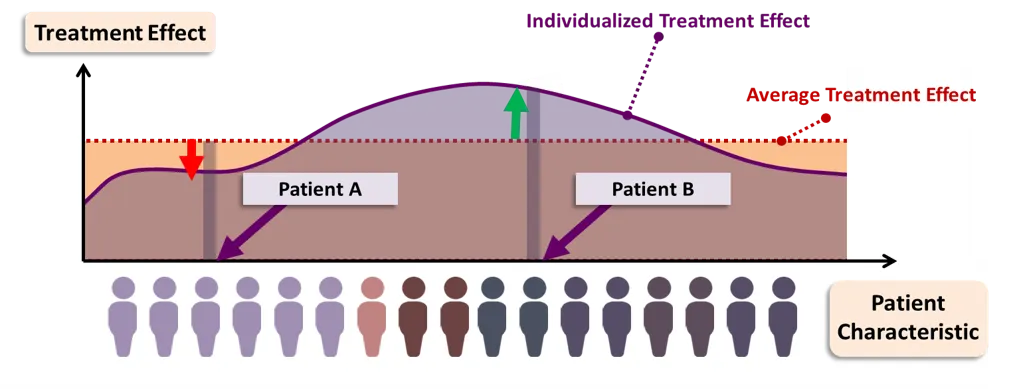
Practitioner initiatives
- Independent drug review journals by HC practitioners for HC practitioners. The largest is the International Society of Drug Bulletins. They perform manual and limited statistical validation of clinical trial data published by pharmaceutical companies.
- Practitioner-led Quality-of-Care data collection initiatives, for example DICA in the Netherlands. They are looking to build cross-institutional understanding of the best practices for administering and executing specific treatments and protocols.Clinical decision support solutions
- Differential privacy & federated learning methods allow HC researchers to collaborate on multi-institution datasets, e.g. FedBioMed.
- Statistical methods to estimate Individual Treatment Effects (ITEs), for example by those developed by Vanderschaar Lab.
- The adoption of EHR data API standards such as FHIRv5.
Clinical decision support solutions
- Atropos Health. Founded in 2020, EUR 13M (USD 14M) in funding to date. This is one of the medical AI startups I’m most excited about — they focus on building digital twins of patients using the millions of EHR records they have in their databases through collaborations with different hospitals and other HC institutions. They use these digital twins to predict the responses of individual patients to different kinds of medications (the ITE, see above), giving clinicians the option to get a second opinion leveraging millions of datapoints.
AI drug discovery solutions
- I didn’t really dive into this area, but check out this blogpost for a listing of some notable startups, or this McKinsey write-up for an outline of the general trend. It’s still early days, but there are quite a few AI startups out there already pulling their weight — expect this to increase with new advances in precision medicine. Drug discovery is also one of the few areas in HC where companies and researchers can leverage all the benefits of AI and compute without much of the risk involved in making real-world decisions that directly affect patient health and lives.
One more thing that could potentially alleviate the existing data dependency on pharmaceutical companies (and I haven’t heard much talk of this, but I’m also not an industry insider), would be for institutions like the EMA in Europe and FDA in the US to clinically reproduce 1 out every 10 studies submitted to them. Additional legislative measures could include mandatory 1, 2, and 5 year follow-up studies on drug effectiveness and side-effects to be performed by pharmaceutical companies after a new drug has been brought to market.
Problem #3: Network effects
As we saw in the introduction with the example of GPT4 outperforming human ophthalmologists on medical question answering with quite a wide margin, it is not a controversial claim that in 2023 (and beyond) humans are worse than Large Language Models (LLMs) at rote memorisation. There are plenty of other studies out there showing LLMs beating humans in most disciplines in terms of both the depth and breadth of knowledge they can store in their model weights, and the efficacy of their ability to recall this knowledge. The problem is that whereas for many other disciplines rote memorisation was an important part of the curriculum, in medicine it has been the central pillar for several thousand years, and continues to be up to this day — medicine has to a large extent been an oral tradition since the shamans of old invoking animal spirits to cure their patients of the dark energy that (reportedly) consumed them. It is human nature that, when we don’t understand something, we make up stories that give us the illusion of control (and the confidence to face others). This is particularly true for medicine, which is just barely scratching the surface of the complexity of the individual components making up the human body from the cellular level on up. Let alone that we know of how the whole thing fits together.
This means that how HC professionals see the human body, how they see patients, and how they practice medicine is to a large extent determined by what their peers and predecessors think and do. Whether it is through informal dinners, conferences or when guiding new physicians in training during their internship, peers fill the gaps of clinical science with a status quo of established practices, protocols and idiosyncrasies. This way of teaching medicine is not only incredibly inefficient (when was the last time your mentor opened a textbook or updated his or her knowledge?), it can also be actively harmful both for patient outcomes and for student mental health. Some steps are being taken to alleviate the current situation and introduce new AI tools, but the overall impression I get is that the inertia stemming from the established medical practice is quite big — not strange of course, given how the current crop of physicians has had to invest so many years in the existing system that they have had no choice but to adopt its core values or drop out.
I noticed one side-effect of this way of working while doing research for my master thesis many many years ago. I was working with pharmacy survey data from the Netherlands, which showed that each year a different drug would take the number one spot in terms of number of prescriptions written out by GPs in the Netherlands (one especially troublesome drug in this category are statins, which are often prescribed to alleviate symptoms of an unhealthy lifestyle). These prescription statistics didn’t match any epidemiological data — this means that the ‘default’ GP prescription for any of those given years I had in the dataset wasn’t based on solid evidence or measured benefit for the patient. What is worse, these prescriptions can often can cause very real side-effects, no drug is completely harmless. It stuck with me all these years as a prime example of one the main issues with modern medicine — the randomness with which GPs switch between prescriptions. Furthermore, if a GP can be swayed this easily by peers (who might not have any ulterior motives), it is also means they can be susceptible to influence by marketing from pharmaceutical companies with profit-maximising motives.
To be clear, I don’t suspect any of this happens through wilful negligence — in fact, many physicians today are painfully aware of the shortcomings of their current way of working. The reality is that the amount of new treatments and healthcare protocols, drugs and clinical studies being published each week makes it practically impossible for HC practitioners to keep up with the latest data and generate their own informed opinions, even when they specialise in a subdomain. I’ve talked to physicians who admitted that spending half their working week updating their knowledge wouldn’t be enough to keep up with everything that is going on in the areas of medicine relevant to them. On top of this, there are many physicians and other HC practitioners who don’t really find the idea of studying each night every night for hours on end until the day they retire just to keep up very appealing — and who can blame them?
Fortunately there are more and more voices in medicine advocating for a wider adoption of AI solutions in medicine to alleviate some of these issues. One of the most prominent of those is Dr. Eric Topol, whose books and substack I can readily recommend. A summary of his views can also be found in this paper published in Nature outlining a general framework for LLM adoption in medicine. I’ve listed some other practitioner initiatives and startups working on solving this issue below.
Practitioner knowledge sharing initiatives
- The Human Diagnosis Project pools combined medical knowledge and uses AI for information retrieval. If you are a programmer, this is like a StackOverflow (or these days, GPT4) for clinicians.
- Networking events like conferences and dinners help practitioners keep their knowledge up to date — or at least in sync with their peers.
- Independent drug review journals by HC practitioners for HC practitioners.
- Search engines for HC practitioners like Artsportaal in the Netherlands (recently acquired by Springer Media).
Content publishers
- Elsevier’s ClinicalKey. An information retrieval tool that lets HC professionals search content from selected Elsevier journals and textbooks for conditions, drugs, dosage etc.
GenAI startups
- Suki.ai. Are upgrading their AI voice assistant to allow for in-consult EHR information retrieval and medical question answering (mQA) based on best practices. I'm not sure exactly how they are going to curate their data, best reach out to them directly for more information.
- Glass Health. Founded in 2021, raised ~EUR 7M (USD 7M) to date. Retrieval Augmented Generation (RAG) combining LLMs with a curated clinical knowledge database maintained by HC professionals.
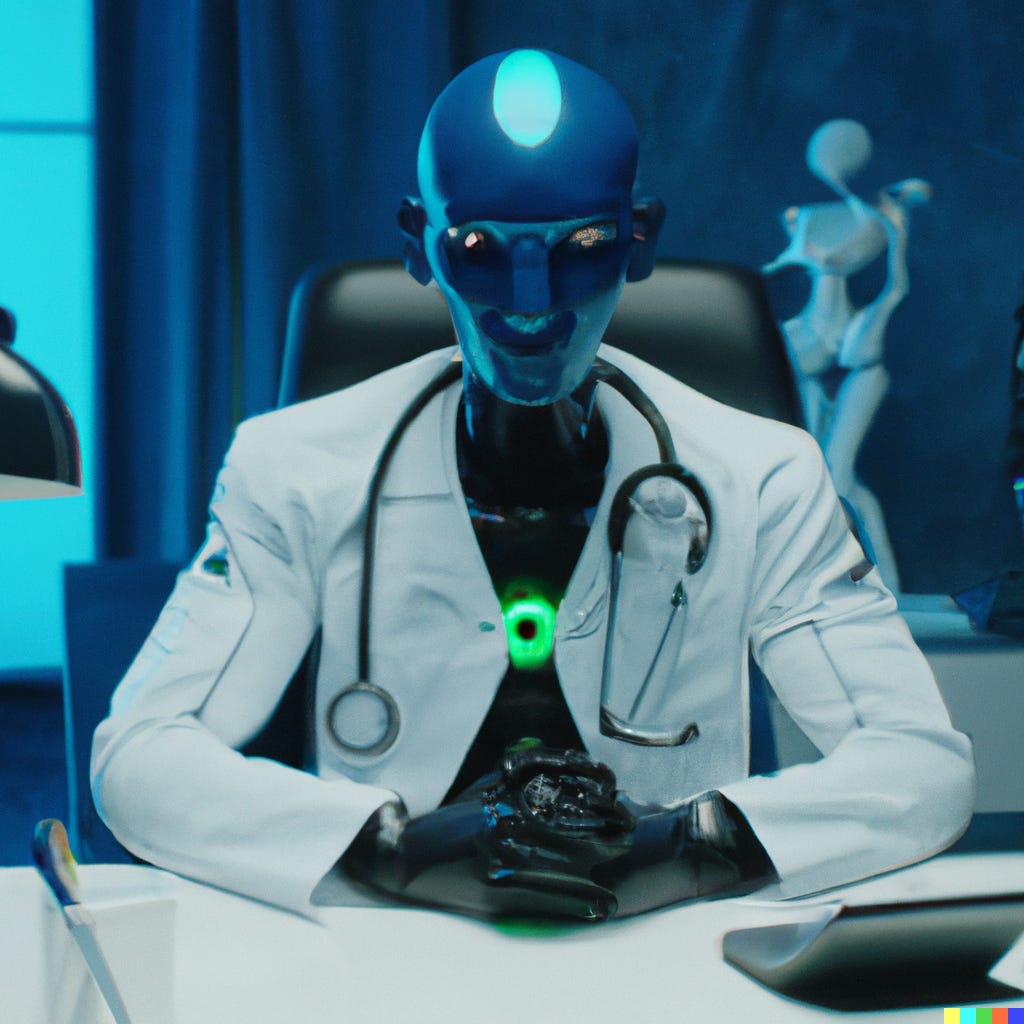
Your AI-augmented physician will see you now
The scale of this problem in terms of human lives impacted and economic costs makes it hard to imagine change of any kind (the EU spent an estimated ~1.5 trillion euros on healthcare in 2020, the US an estimated ~4.2 trillion dollars). At the same time it is also one of the areas where adopting AI solutions and data best practices can truly make a difference. For AI, it would certainly be a big step up from optimising online ads. The risks are of course higher — and any AI system is probabilistic in nature so these risks need to be managed appropriately — but as I hope I’ve been able to show, there is also ample room for improvement. Given how poorly data is currently being managed across healthcare, just getting institutions and systems to step up their data collection and auditing game would already be a big leap forward for medicine and mankind as a whole.
A key part in managing AI implementation risks will be to build the right AI software solutions and provide HC practitioners with the right training. This includes familiarising them with the limitations inherent in any probabilistic reasoning engine, but also showing them the opportunities these systems bring to augment the current medical practice and automate those parts of the work where software outperforms humans.
A final disclaimer — I am not a medical professional, nor have I ever worked in the healthcare industry. All of my opinions and ramblings in this blog are the result of a mere three weeks of research I did to see if there was any life in the startup idea I had (an AI assistant for GPs), so I probably missed some of the finesse that comes with actually working in the industry. I ended up abandoning that startup idea seeing that there were people more qualified than myself working on most of the key ideas I had for this AI assistant — I’m 100% rooting for Atropos Health and Suki.ai! That being said, I’m always open to learn and happy to stand corrected.
If you enjoyed this write-up and want to read more of my ramblings about AI, data, society and the economy, sign up to my newsletter below!
Member discussion